Dynamic Spatio-Temporal Graph Convolutional Networks
Author(s)
Tell, Max R.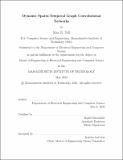
DownloadThesis PDF (834.4Kb)
Advisor
Mazumder, Rahul
Terms of use
Metadata
Show full item recordAbstract
Spatio-temporal modeling is an essential lens to understand many real-world phenomena from traffic [20] [10] to epidemiology [12]. Although forecasting time series is an exceptionally well-studied problem, recent years have seen impressive gains in the performance of graph learning as a paradigm for spatial learning problems. Some recent work has explored the intersection of these two fields but often assumes that the underlying graph structure is static. We introduce Dynamic Spatio-Temporal Graph Convolution Network (DST-GCN) as a novel architecture for spatio-temporal modeling with changing graph structure. DST-GCN employs a convolutional architecture to learn spatio-temporal relationships that provide strong generalization and attractive computational efficiency. We provide empirical results for several datasets from different domains that demonstrate the gains provided by DST-GCN.
Date issued
2022-05Department
Massachusetts Institute of Technology. Department of Electrical Engineering and Computer SciencePublisher
Massachusetts Institute of Technology