Smart Remote Personal Health Monitoring System: Addressing Challenges of Missing and Conflicting Data
Author(s)
Zhu, Ye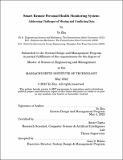
DownloadThesis PDF (3.832Mb)
Advisor
Gupta, Amar
Terms of use
Metadata
Show full item recordAbstract
Clinical usage of Remote Patient Monitoring (RPM) systems has spurred during the past two years. Driven by an increase in demand during the COVID-19 pandemic, Internet of Medical Things (IoMT) systems are becoming much more diverse and prevalent. They are excellent candidates for monitoring patients’ health status and disease state, for predicting patients’ response to treatment, for alerting extraordinary, acute, or emergency events, for analyzing and managing large datasets, and for preventing disease progression and symptom manifestation.
Although healthcare technology had improved over the years, two main challenges to enhance remote patient monitoring or improve professional telehealth programs continue to be interoperability and data handling. Many new solutions have been under investigation as the pandemic shifts the world’s perspective on how healthcare should be performed to treat acute, chronic, psychological and infectious diseases.
This thesis first focuses on using a system thinking approach to design and architect a low-cost and scalable RPM system for general applications. The key challenges and potential solutions are discussed from the system design and architecture points of view.
To deep dive into particular data challenges faced by researchers implementing big-data analytics for remote monitoring, the second part of this thesis selects the remote smart cardiac health monitoring system as the example system for detail technical aspect analysis. Several deep learning methods, including RNN, BRITS, GAN, DeepAR based methods, are applied to address the missing data issues during remote monitoring,. The methods are tested and compared to each other. A federated learning approach is also explored and proposed to be implemented in distributed remote patient monitoring systems for improving privacy and security.
Date issued
2022-05Department
System Design and Management Program.Publisher
Massachusetts Institute of Technology