Application of Unsupervised Machine Learning for Event Classification
Author(s)
Kryhin, Serhii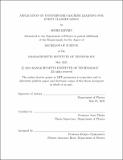
DownloadThesis PDF (2.104Mb)
Advisor
Thaler, Jesse
Terms of use
Metadata
Show full item recordAbstract
We study quark and gluon jets separately using public collider data from the CMS experiment. Our analysis is based on an Open Data dataset of proton-proton collisions collected at the Large Hadron Collider in 2011. We define two non-overlapping data mixtures via a pseudorapidity cut—central jets with |𝜂| ≤ 0.65 and forward jets with |𝜂| > 0.65—and employ jet topic modeling to extract individual distributions for the maximally separable categories. Under certain assumptions, such as sample independence and mutual irreducibility, the extracted “topic” categories correspond to “quark” and “gluon” distributions. We consider a number of different methods for extracting reducibility factors from the central and forward datasets and determine fractions of quark jets in each sample dataset. We also utilize the extracted fractions to reconstruct the distributions of observables for “quark” and “gluon” components, explore the change of topic fraction with the rapidity spectrum, compute the intrinsic dimensionality for each of the topics, and perform a crosscheck by exploring the tagging performance. The greatest stability and robustness to statistical uncertainties is achieved by a novel method based on parametrizing the endpoints of a receiver operating characteristic (ROC) curve. To mitigate detector effects, which would otherwise induce unphysical differences between central and forward jets, we use the OmniFold method to perform central value unfolding. To our knowledge, this work is the first application of full phase space unfolding to real collider data, and one of the first applications of topic modeling to extract separate quark and gluon distributions at the LHC.
Date issued
2022-05Department
Massachusetts Institute of Technology. Department of PhysicsPublisher
Massachusetts Institute of Technology