Bio-Signal Analysis for Personalized Pilot Training
Author(s)
Powell, Stuart D.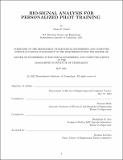
DownloadThesis PDF (28.44Mb)
Advisor
Heldt, Thomas
Rao, Hrishikesh M.
Terms of use
Metadata
Show full item recordAbstract
Quantitative data on pilot performance can help increase efficiency in pilot training programs by informing when students are prepared take on more difficult challenges. Previous research indicates attentional and cognitive load differences in novice pilots versus more experienced pilots. These differences may manifest as changes in certain physiological signals as pilots of differing experience levels are over, under, or adequately challenged in a given task. This thesis analyzes the effectiveness of measured elecrodermal activity (EDA) and electromyography (EMG) signals to indicate trends in pilot experience, task performance, and challenge difficulty across N = 29 subjects.
EMG and EDA features, as well as accelerometry and joystick data, are considered over the entire task and shorter windows in at the beginning and end of each task. Significant differences, with a p-value less than 0.05, are seen in EMG features based on difficulty, experience, and trial attempt and in EDA features based on experience and trial attempt.
Using these features, tasks by a subject are classified using a logistic regression model with forward step-wise feature selection. The performance of the model in classifying the easiest against the hardest difficulty level reaches an AUC of 0.99, reduced to 0.83 with the dominant joystick feature removed. Classifying a run as performed by an expert against a novice (using a cutoff of 30 flight hours) in the hardest difficulty level reaches an AUC of 0.89, reduced to 0.67 with the dominant joystick data removed. Lastly, the model performance when classifying the first against the last attempt of a subject at a given difficulty level reaches an AUC of 0.85 across all subjects.
Date issued
2022-05Department
Massachusetts Institute of Technology. Department of Electrical Engineering and Computer SciencePublisher
Massachusetts Institute of Technology