Analyzing Student’s Problem-solving Approaches in MOOCs using Natural Language Processing
Author(s)
Kong, ByeongJo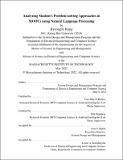
DownloadThesis PDF (4.425Mb)
Advisor
O’Reilley, Una-May
Hemberg, Erik
Terms of use
Metadata
Show full item recordAbstract
Problem-solving processes are an essential part of learning. Knowing how students approach solving problems can help instructors improve their instructional designs and effectively guide the learning process of students. This thesis proposes a natural language processing (NLP) driven method to capture online learners’ problem-solving approaches while using Massive Open Online Courses (MOOCs) as a learning platform. It employs an online survey to gather data, NLP techniques, and existing educational theories to investigate this in the lens of both computer science and education.
The thesis considers survey responses from students enrolled in a computer programming course taught on edX in Spring 2021. A total of 7,482 free-text responses are selected from 44,864 responses collected through the survey. The thesis shows how NLP techniques, i.e. preprocessing, topic modeling, and text summarization, must be tuned to extract information from a large-scale text corpus. The proposed method discovered 18 problem-solving approaches from the text data, such as using pen and paper, peer learning, trial and error, etc. By using datasets from 2020 and 2021, we also learned that there are strong topics that appear over the years, such as clarifying code logic, watching videos, etc. Lastly, we used existing educational theories to discuss the findings from a viewpoint of education.
Date issued
2022-05Department
Massachusetts Institute of Technology. Department of Electrical Engineering and Computer Science; System Design and Management Program.Publisher
Massachusetts Institute of Technology