Use of machine learning in radio frequency integrated circuits (RFIC) development
Author(s)
Cui, Qiang (Computer engineer).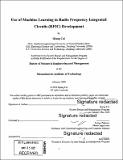
Download1342001380-MIT.pdf (12.90Mb)
Other Contributors
Massachusetts Institute of Technology. Engineering Systems Division.
System Design and Management Program.
Terms of use
Metadata
Show full item recordAbstract
This Master's Thesis starts with an introduction to the radio frequency integrated circuits (RFICs) industry and a discussion on the key problem of the existing RFIC development process: the need for multiple trial and error iterations due to inaccurate simulations. This simulation inaccuracy happens because the existing electronic design automation (EDA) software, and the underlying physics-based IC models, fail to fully capture the nonlinear, frequency-dependent RF parasitic effects. To overcome this problem, in this thesis we propose the use of machine learning in RFIC development. Machine learning uses statistical models to recognize hidden patterns from sample data points, known as "training"; generalize patterns; and make predictions based on new data. In theory, machine learning can capture the nonlinear, frequency-dependent RF parasitic effects very well thanks to the large variety of nonlinear modelling techniques at its disposal, such as polynomial regressions and neural networks. Therefore, this thesis investigates for the first time the feasibility of using machine learning in RFIC development to solve the problem of inaccurate RFIC simulation. Chapter two describes how to represent and collect the RF and spec data to be able to use them in machine learning. The data needs to be represented in the format of {X: design parameters, Ysim: EDA simulation results, Ytrue: test results}. Ideally, large datasets should be collected by testing fabricated ICs. However, in this thesis we used electromagnetic-enabled mixed mode simulation data as an alternative to actual test data for demonstration purposes. Chapter three summarizes the existing RFIC development flow and describes the three different blocks in the flow where machine learning could be added: (1) between the customer specifications and the circuit design, or specs-to-design; (2) between EDA simulation and circuit fabrication, or simulation-to-fabrication; and (3) between lab test results and design revision, or test-to-re-design. Chapter four studies each block design level in detail by applying two basic machine learning techniques: polynomial regression (PR) and neural networks (NN). Chapter five provides case studies for developing RF switches using machine learning. The results show that machine learning can significantly improve the prediction accuracy, which proves the feasibility of using machine learning in RFIC development. The research developed in this Thesis has strong potential to impact the RFIC industry. Unlike digital circuit design where the high accuracy of EDA simulations allows for highly automated circuit development, the RFIC design industry suffers from significant simulation inaccuracies. Hence, RFIC development typically requires multiple time-consuming and costly design-fabrication iterations. Some researchers have already used machine learning to improve the step between the initial specifications and design, but those solutions are not really effective because of their large computational complexity. Those researchers in ran hundreds (if not thousands) of simulations using existing EDA tool, and used those simulations to train neural network model so the model can learn how to design circuit. The hundreds of simulations cause the computational complexity. In many cases circuit designs using these techniques take even longer time than existing solutions in the industry. In contrast, this thesis focuses on the use of machine learning to optimize block #2, that is between simulation and fabrication to provide accurate predictions. The task of accurate prediction in this thesis needs less computation resource but provides more helpful to RFIC development. This thesis shows that the simulation accuracy can be improved by 98%, which will dramatically reduce the need for multiple design-fabrication iterations. This improvement means significant time and cost reduction in RFIC products.
Description
Thesis: S.M. in Engineering and Management, Massachusetts Institute of Technology, Engineering Systems Division, System Design and Management Program, 2020 Cataloged from PDF version of thesis. Includes bibliographical references (page 50).
Date issued
2020Department
Massachusetts Institute of Technology. Engineering Systems Division; System Design and Management Program.Publisher
Massachusetts Institute of Technology
Keywords
Engineering Systems Division., System Design and Management Program.