Cause, Composition, and Structure in Language
Author(s)
Qian, Peng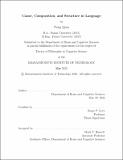
DownloadThesis PDF (3.143Mb)
Advisor
Levy, Roger P.
Terms of use
Metadata
Show full item recordAbstract
From everyday communication to exploring new thoughts through writing, humans use language in a remarkably flexible, robust, and creative way. In this thesis, I present three case studies supporting the overarching hypothesis that linguistic knowledge in the human mind can be understood as hierarchically-structured causal generative models, within which a repertoire of compositional inference motifs support efficient inference. I begin with a targeted case study showing how native speakers follow principles of noisy-channel inference in resolving subject-verb agreement mismatches such as "The gift for the kids are hidden under the bed". Results suggest that native-speakers' inferences reflect both prior expectations and structure-sensitive conditioning of error probabilities consistent with the statistics of the language production environment. Second, I develop a more open-ended inferential challenge, completing fragmentary linguistic inputs such as "____ published won ____." into well-formed sentences. I use large-scale neural language models to compare two classes of models on this task: the task-specific fine-tuning approach standard in AI and NLP, versus an inferential approach involving composition of two simple computational motifs; the inferential approach yields more human-like completions. Third, I show that incorporating hierarchical linguistic structure into one of these computational motifs, namely the auto-regressive word prediction task, yields improvements in neural language model performance on targeted evaluations of models’ grammatical capabilities. I conclude by suggesting future directions in understanding the form and content of these causal generative models of human language.
Date issued
2022-05Department
Massachusetts Institute of Technology. Department of Brain and Cognitive SciencesPublisher
Massachusetts Institute of Technology