A Predictive Model for Pancreatic Cancer Diagnosis
Author(s)
Xiong, Thomas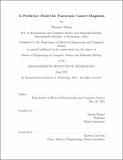
DownloadThesis PDF (8.994Mb)
Advisor
Rinard, Martin
Terms of use
Metadata
Show full item recordAbstract
Pancreatic ductal adenocarcinoma (PDAC), a specific type of pancreatic cancer, has a five-year survival rate of 8.5% and is the third-deadliest cancer in the United States. However, earlier detection can raise survival rates dramatically. In this thesis, we investigate the hypothesis that predictive models from a variety of model classes can use different indicators from electronic health record (EHR) data in order to predict PDAC diagnosis. We find that logistic regression, random forest, and XGBoost models perform the best when using patients’ unique diagnoses, lab test frequencies, medication frequencies, and race and ethnicity as data, with our best logistic regression model achieving an AUROC of 0.801 on a held-out test set. To better approximate these models’ use case in practice, we construct a time-dependent regime for model evaluation. Overall, we found that model performance decreased in the time-dependent regime as compared to the time-independent regime, suggesting the possibility of concept drift in our dataset. Moreover, through ℓ₀ regularization, we found that lab test frequencies tended to be the most important features in the best logistic regression model. The intended use for our deployed model is to serve as a prescreening tool to deliver an enriched population for further targeted PDAC screening. Our best model for this purpose delivers a sensitivity of 0.46 at a specificity of 0.9. According to our medical collaborators, this combination of sensitivity and specificity qualifies this model as suitable for our intended prescreening use. In this context, the ability of our model to work only with information derived from electronic health records, collected as part of routine medical care, is a significant advantage. We describe the steps taken to begin to model deployment into an existing federated EHR database. In this scenario, we envision that our model would be integrated into hospital EHR systems and routinely and automatically run over broad patient populations as EHR data is collected over time to produce a history of patient risk scores as patient data becomes available. Patient selection for further targeted PDAC screening can then consider both absolute scores and their evolution.
Date issued
2021-06Department
Massachusetts Institute of Technology. Department of Electrical Engineering and Computer SciencePublisher
Massachusetts Institute of Technology