Differentiable Multiscale Molecular Simulations
Author(s)
Wang, Wujie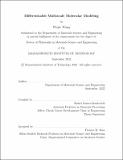
DownloadThesis PDF (15.75Mb)
Advisor
Gómez-Bombarelli, Rafael
Terms of use
Metadata
Show full item recordAbstract
Multiscale molecular simulation is a critical tool to understand matter. The multiscale picture of simulations views observables at different granularities, providing explanation and prediction of phenomena across a wide range of spatial and temporal scales. Recently, data-driven modeling has shown great success in improving the predictive powers of molecular simulations from modeling of electronic structures to macroscopic phenomenology. Much of the success is built on deep and end-to-end differentiable models trained on high-quality big datasets with gradient-based optimizations. To fully exploit the power of data-driven multi-scale simulations, this thesis explores the application of differentiable algorithms on multiscale molecular modeling. Specifically, I introduce algorithms in three problem domains where differentiable modeling shows great promises: 1) differentiable graph-based force field construction for multi-scale molecular simulations; 2) end-to-end differentiable molecular dynamics for learning and control based on coarse-grained observables; 3) differentiable and generative scale-hopping between fine-grained and coarse-grained dynamics. The algorithms introduced in this thesis bridge the gap between scales for data-driven modeling, opening possibilities for more powerful and predictive multiscale models.
Date issued
2022-09Department
Massachusetts Institute of Technology. Department of Materials Science and EngineeringPublisher
Massachusetts Institute of Technology