Machine Learning Applications For Neurological Diseases
Author(s)
Gold, Maxwell P.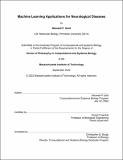
DownloadThesis PDF (21.99Mb)
Advisor
Fraenkel, Ernest
Terms of use
Metadata
Show full item recordAbstract
Neurological conditions affect the brain and other parts of the nervous system. This includes neurodegenerative diseases like Huntington’s Disease, psychiatric conditions like schizophrenia, and brain cancers like glioblastoma. These conditions are particularly challenging to study because they affect such a vital and complex organ system, making it difficult to understand disease etiology and to develop high-quality model systems.
Because of these challenges, experiments studying neurological diseases typically either contain very few patient samples or are collected from imperfect model systems. Machine learning approaches have proven helpful for processing these types of datasets and identifying relevant biological signal. In this thesis, I detail five examples of the utility of machine learning methods for analyzing neurological disease data. Some chapters focus primarily on the development of novel machine learning methods, while others discuss the implementation of established algorithms leading to significant advancements in our understanding of the given disease.
Chapter 2 details a novel gene set scoring algorithm that significantly improves upon existing methods. This new approach is particularly useful for analyzing single-cell transcriptomics assays, which are becoming increasingly common in neurological disease studies. In Chapter 3, I describe how multi-omic integration of ATAC-Seq, ChIP-Seq, and RNA-seq data revealed a novel population of cycling cells relevant to Huntington’s Disease models. In Chapter 4, I discuss an improved multi-commodity flow algorithm for omics data integration and highlight its utility for understanding drug effects in glioblastoma. Chapter 5 highlights how clustering and the Prize-Collecting Steiner Forest algorithm led to a better understanding of proteomic subtypes in medulloblastoma tumors. Lastly, Chapter 6 expands upon the work in Chapter 5, and details how I used computational approaches to figure out that some medulloblastoma tumors contain cells recapitulating cerebellar granule neuron development.
In summary, this thesis showcases the value machine learning techniques for analyzing the small, complicated datasets typically found in neurological disease experiments. Throughout this work, I emphasize the importance of collecting and integrating multiple types of biological data to get a more complete understanding of these conditions.
Date issued
2022-09Department
Massachusetts Institute of Technology. Computational and Systems Biology ProgramPublisher
Massachusetts Institute of Technology