Hybrid Concurrent Planning with Heterogeneous Robot Teams for Timed Goals
Author(s)
Chen, Jingkai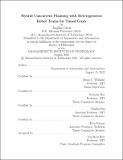
DownloadThesis PDF (21.45Mb)
Advisor
Williams, Brian C.
Terms of use
Metadata
Show full item recordAbstract
Robotic techniques in terms of mechanical design, perception, and planning have been improving at a dramatic rate. However, these advances for single autonomous systems will not be sufficient for many real-world applications, in which robots need to collaboratively complete tasks together given their different capabilities, while avoiding conflicts in terms of competing energy resources and occupied space, such as a cooperative truck-and-drone delivery system and smart warehouse. In these scenarios, we need not only to assign timed goals to robots but also plan all the robots to achieve these goals.
Planning and coordinating for robot teams to complete multiple timed goals in a long horizon is challenging: (1) reasoning over hybrid systems with both discrete and continuous specifications; (2) coordinating multiple robots and timed goals; (3) optimizing towards high-quality solutions. As a result, the solution space of this problem is huge and features combinatorial and nonlinear behaviours, and finding feasible solutions is nontrivial not to say finding high-quality ones.
There are two streams of research that address this problem: (1) hybrid activity planning, which plans both the symbolic and numeric parts of a system; (2) multirobot motion planning, which targets at coordinating collision-free motions of a large fleet of vehicles. While the first line of research mainly considers limited numeric parts, multi-robot motion planning lacks the ability to reason over high-level activities. Few of them can completely solve our problems.
In this thesis, I address this problem by adopting a two-stage hierarchical planning framework, which combines the strengths of both lines of research mentioned above. This planning framework divides the planning procedure into two stages: (1) highlevel hybrid activity planning: optimally plan the activities of all the robots by using centralized algorithms, while partially considering robot dynamics, such as approximating nonlinear dynamics to be linear; (2) low-level multi-robot motion planning in support of activities and deadlines: given the planned activities, plan safe, executable control trajectories of all the robots in a decoupled way.
This two-stage design is efficient, while being reasonably effective and complete: (1) to be efficient, the high-level planner properly approximates robot dynamics, and the low-level planner decouples the planning problem to single-activity subproblems and only coordinates them on demand; (2) the high-level planner is guaranteed to generate optimal decisions over activities and the low-level planner grounds these planned activities with an optimization purpose; (3) to mitigate the incompleteness due to the hierarchy, we provide rich information for making high-level decisions.
Under this framework, this thesis has three major contributions: (1) an optimal hybrid activity planner, cKongming, that represents all the possible robot trajectories as a temporal hybrid flow graph and further encode and solve as a Mixed Integer Linear Program (MILP). Key to cKongming’s efficiency and effectiveness is its graph representation, which combines the hybrid flow graphs of the PDDL-K planner Kongming, to be effective, and the adaptive-duration action representation in PDDL 2.1 planners, to be efficient. (2) a scalable, effective multi-robot motion coordinator for activity plans, which extends the priority-based single-goal coordination that coordinates by specifying priorities between agents, to handle multiple activities with temporal constraints. Key to achieving this is to specify priorities between individual activities, rather than the complete activity plans of different robots. (3) an assembly planner that automatically plans multiple high-dimensional manipulators for assembly tasks. The planner is built under the same two-stage hierarchical planning framework. The activity planner simplifies the hybrid planner to a temporal planner and leverages an analogy to solving Vehicle Routing Problem with Time Windows to achieve efficiency. The task-directed motion planner tailors our general motion coordinator by allowing more aggressive single-activity replanning and look-ahead for future reachability. In the demonstration, we can plan assembly plans for up to three robots and 23 objects in a couple minutes.
Date issued
2022-09Department
Massachusetts Institute of Technology. Department of Aeronautics and AstronauticsPublisher
Massachusetts Institute of Technology