Analysis of Continuous Tensor-Train Methods for Optimal Control Problems with the Ornstein-Uhlenbeck Operator
Author(s)
White, Joshua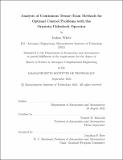
DownloadThesis PDF (2.456Mb)
Advisor
Marzouk, Youssef M.
Terms of use
Metadata
Show full item recordAbstract
Continuous tensor-train decompositions methods have been found to well approximate optimal control policies under certain conditions for dynamic programming problems within the Compressed Continuous Computation (C3) framework. We aim to utilize numerically approximated control solutions found using such tensor-train methods as importance functions for adaptive multilevel splitting algorithms to investigate rare event probabilities related to stochastic dynamical systems and thereafter evaluate the variances and relative errors of the resulting statistical estimators. We also evaluate the efficacy of Compressed Continuous Computation in solving time-dependent Hamilton-Jacobi-Bellman partial differential equations for a limited class of systems whose aforementioned solutions are exactly known by calculating point-wise squared deviations and L2 errors between computed and analytical solutions. We find that Compressed Continuous Computation produces fruitful importance functions for adaptive multilevel splitting algorithms, resulting in probability estimators with significant variance and relative error reduction, however we additionally find that due to significant numerical error the C3 framework is not suitable for accurately solving time-dependent partial differential equations.
Date issued
2022-09Department
Massachusetts Institute of Technology. Department of Aeronautics and AstronauticsPublisher
Massachusetts Institute of Technology