Quantized Guessing Random Additive Noise Decoding - A Universal Quantized Soft-Decoder
Author(s)
Gabhart, Evan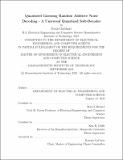
DownloadThesis PDF (336.0Kb)
Advisor
Médard, Muriel
Duffy, Ken R.
Terms of use
Metadata
Show full item recordAbstract
Guessing Random Additive Noise Decoding (GRAND) has proven to be a universal, maximum likelihood decoder. Multiple extensions of GRAND have been introduced, giving way to a class of universal decoders. GRAND itself describes a hard-detection decoder, so a natural extension was to incorporate the use of soft-information. The result was Soft Guessing Random Additive Noise Decoding (SGRAND). SGRAND assumes access to complete soft information, proving itself to be a maximum-likelihood soft-detection decoder. Physical limitations, however, prevent one from having access to perfect soft-information in practice.
This thesis proposes an approximation to the optimal performance of SGRAND, Quantized Guessing Random Additive Noise Decoding (QGRAND). I describe the algorithm and evaluate its performance compared to hard-detection GRAND, SGRAND, and another approach to approximating SGRAND, Ordered Reliability Bits GRAND (ORBGRAND). QGRAND also allows itself to be tailored to an arbitrary number of bits of soft information, and I will show as the number of bits increases so does performance. I then use the GRAND algorithms discussed in order to evaluate error correction potential of different channel codes, particularly Polar Adjusted Convolutional codes, CA-Polar codes, and CRCs.
Date issued
2022-09Department
Massachusetts Institute of Technology. Department of Electrical Engineering and Computer SciencePublisher
Massachusetts Institute of Technology