Machine Learning Approaches for Equitable Healthcare
Author(s)
Chen, Irene Y.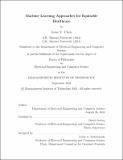
DownloadThesis PDF (2.110Mb)
Advisor
Sontag, David
Terms of use
Metadata
Show full item recordAbstract
With the proliferation of clinical data and algorithms to improve clinical care, researchers are increasingly concerned about the equity and fairness of the resulting machine learning models. Because the observational data we collect can be noisy, incomplete, and biased, seemingly straight-forward implementation of existing methods for clinical intervention or better understanding human knowledge can lead to inaccurate and inequitable clinical algorithms. To begin to address these challenges, we need new tools to tackle the bias that can arise when modeling data. In this work, we present machine learning approaches for auditing, ameliorating, and preventing bias in the machine learning for healthcare model development process. In particular, we focus on case studies that can provide actionable insights.
In this thesis, we present several examples of machine learning approaches towards equitable healthcare and recommend changes based on the results of the corresponding experiments. Questions of equity and bias can be thought of in terms of the different steps of the model development pipeline. We argue that these model development steps can be made more equitable and unbiased when they 1) mitigate algorithmic bias that may occur from biased data collection or model development, and 2) address known existing systemic health disparities.
We present four case studies of machine learning approaches towards equitable healthcare, and demonstrate these approaches on real clinical tasks. First, we decompose the sources of discrimination and provide empirical estimation techniques. We present results on applying these techniques in the task of intensive care unit mortality prediction and salary prediction. Second, we consider the predictive analytics of health insurance providers, namely predicting the likelihood of hospitalization and the likelihood of high-risk pregnancy. We apply the same discrimination decomposition techniques towards practical steps for mitigating algorithmic discrimination. Third, we study the task of clustering interval-censored time-series data. We develop a deep generative model, called SubLign, to learn the latent delayed entry alignment value for each time-series as well as the heterogeneous progression patterns across the population. We evaluate our model in the context of synthetically generated data. Following, we study the task of disease subtyping for the improved understanding of disease progression. We present results on clustering clinical patients including heart failure and Parkinson’s disease. Finally, we study an example of using machine learning on an understudied problem that affects underserved patients: early detection of intimate partner violence. We develop a model that predicts the likelihood of eventual intimate partner violence self-reporting and radiology injury labeling from radiology reports. We conclude with a discussion about how machine learning can continue to address equity and bias in healthcare.
Date issued
2022-09Department
Massachusetts Institute of Technology. Department of Electrical Engineering and Computer SciencePublisher
Massachusetts Institute of Technology