Optimizing Healthcare Delivery in Resource-Limited Settings
Author(s)
Gibson, Emma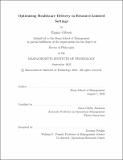
DownloadThesis PDF (10.33Mb)
Advisor
Jónasson, Jónas Oddur
Terms of use
Metadata
Show full item recordAbstract
In resource-limited settings, critical diagnostic testing services are frequently provided through hierarchical networks comprised of healthcare facilities that collect diagnostic samples (e.g. blood, nasal swabs) from patients, and centralized medical laboratories that analyze these samples. The first part of this thesis focuses on diagnostic sample transportation systems, which are used to move samples and test results between various locations within centralized networks.
In Chapter 2, we describe the design and implementation of a low-cost information sharing system which allows healthcare workers to report daily sample volumes at each facility within the network using a simple text-based interface accessible on any standard mobile phone. The feasibility and effectiveness of this system were assessed in a field trial at 51 healthcare facilities in Malawi, which achieved high rates of participation and accuracy.
In Chapter 3 we propose an optimized sample transportation system which uses data reported by healthcare facilities to generate efficient routes for sample couriers on a daily basis. This system was implemented in three districts in Malawi, where it reduced average transportation delays by 25% and decreased the proportion of unnecessary trips by 55%.
In Chapter 4 we evaluate operational strategies for the deployment of Point-of-Care (POC) testing at healthcare facilities in Malawi. We develop a mixed-integer model to optimize the allocation of POC instruments to strategic locations within the diagnostic network in order to maximize the benefits of Viral Load monitoring services for people living with HIV. Our analysis indicates that the most cost-effective POC deployment policies include a combination of targeted POC testing of high-risk patients, as well as capacity-sharing strategies such as near-POC testing.
In Chapter 5, we study survival analysis models, which are frequently used to analyze health outcomes and identify risk factors associated with morbidity and mortality. We present a new Globally Optimized Survival Trees algorithm that leverages mixed-integer optimization and local search techniques to generate interpretable survival tree models. We demonstrate that this algorithm improves on the accuracy of existing survival tree methods, particularly in large datasets.
Date issued
2022-09Department
Massachusetts Institute of Technology. Operations Research Center; Sloan School of ManagementPublisher
Massachusetts Institute of Technology