Learning Large-scale Multi-agent Control with Safety Certificates
Author(s)
Qin, Zengyi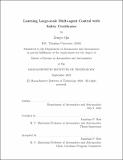
DownloadThesis PDF (3.947Mb)
Advisor
How, Jonathan P.
Terms of use
Metadata
Show full item recordAbstract
Multi-agent intelligence in autonomous systems has been fascinating roboticists for decades. The recent advances in machine learning has created unprecedented opportunities for achieving ultimate multi-agent intelligence and full autonomy in a data-driven way. However, a fundamental bottleneck of machine learning-based methods is their safety and reliability in controlling the autonomous system at large scale, due to the lack of formal safety guarantee. In addressing these challenges, we develop: (1) An machine learning-based large-scale multi-agent control framework with safety certificates, which simultaneously enjoys the versatility of machine learning and the assurance of safety. (2) A multi-agent trajectory tracking framework with convergence and safety guarantees. (3) A general method to learn safe controllers for black-box systems with unknown dynamics. Comprehensive experiments have shown that the proposed methods have notable performance in terms of safety rate, task completion rate, computational efficiency and large-scale scalability.
Date issued
2022-09Department
Massachusetts Institute of Technology. Department of Aeronautics and AstronauticsPublisher
Massachusetts Institute of Technology