Visual Inertial Odometry with Sparse Deep Learning
Author(s)
Saat, Berke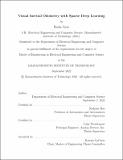
DownloadThesis PDF (19.48Mb)
Advisor
Roy, Nicholas
Prendergast, Colm
Terms of use
Metadata
Show full item recordAbstract
In the field of indoor navigation, visual and inertial sensors are essential to localizing a robot. Visual Inertial Odometry (VIO) systems try to figure out the position and orientation of a robot by analyzing data from cameras and inertial sensors. As state-of-the-art VIO systems achieve remarkable accuracy, there are still improvements to be made in terms of the performance of such real-time systems. This paper presents an end-to-end VIO implementation that combines inertial measurements with wheel velocity information while using visual odometry to further optimize the pose estimates. On the visual odometry side, the paper presents a training module with an automated data collection system and a more sparse graph neural network to train with. We also show how the integration of this deep learning model impacts the performance of a real-time VIO system. On the inertial side, a commonly used sensor like an Inertial Measurement Unit (IMU) has noise and bias, which cause errors that grow fast as they get integrated over time. This paper uses factor graph structures and incremental optimization to minimize these errors and wheel velocity information to stabilize the linear outputs. In the end, we show how each VIO component and each modification to our deep learning model impact the accuracy of our pose estimation and the performance of our end-to-end VIO estimation.
Date issued
2022-09Department
Massachusetts Institute of Technology. Department of Electrical Engineering and Computer SciencePublisher
Massachusetts Institute of Technology