Towards Scalable Structured Data from Clinical Text
Author(s)
Agrawal, Monica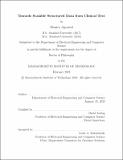
DownloadThesis PDF (4.558Mb)
Advisor
Sontag, David
Terms of use
Metadata
Show full item recordAbstract
The adoption of electronic health records (EHRs) presents an incredible opportunity to improve medicine both at the point-of-care and through retrospective research. Unfortunately, many pertinent variables are trapped in unstructured clinical note text. Automated extraction is difficult since clinical notes are written in their own jargon-heavy dialect, patient histories can contain hundreds of notes, and there is often minimal labeled data. In this thesis, I tackle these barriers from three interconnected angles: (i) the design of human-AI teams to speed up annotation workflows, (ii) the development of label-efficient modeling methods, and (iii) a re-design of electronic health records that incentivizes cleaner data at time of creation.
Date issued
2023-02Department
Massachusetts Institute of Technology. Department of Electrical Engineering and Computer SciencePublisher
Massachusetts Institute of Technology