Untangling the complexity of nature: Machine-learning for accelerated life-sciences
Author(s)
Yaari, Adam U.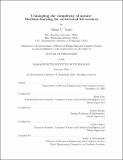
DownloadThesis PDF (30.46Mb)
Advisor
Katz, Boris
Berger, Bonnie
Barbu, Andrei
Terms of use
Metadata
Show full item recordAbstract
The fundamental understanding of living processes is one of the main pillars in modern medicine and technology. Biological mechanisms are convoluted and stochastic systems that remain largely misunderstood despite centuries of rigorous scientific work. In recent years, machine-learning (ML) has resurfaced as a powerful framework to identify patterns of interest in complex datasets. Yet, the impact of such methods remains limited in the broad context of life-sciences. This work optimizes the utility of ML to accelerate research of fundamental biological problems. First, we propose a paradigm shift from siloed data curation to multi-purpose cohorts at scale, even in the most restrictive case of human experimentation. The potential of this approach is revealed through the Brain TreeBank, a multi-modal dataset of naturalistic language aligned to intracranial neural recordings. The TreeBank provides the resolution and breadth required to probe the spatio-temporal dynamics of language context dependence and representation in the brain. Second, we argue for the importance of ML interpretability to accelerate the understanding of biology. We develop an explainable general-purpose tool for modeling discrete stochastic processes at multiple resolutions with output certainty estimation. We demonstrate the utility of the method by modeling patterns of somatic mutations across the entire cancer genome and extend it to map mutation rates in 37 types of cancer. The confidence intervals and increased sensitivity of the method identify sets of mutations that likely drive cancer growth in both coding and noncoding regions of the genome. Broadly, this work demonstrates how computational approaches can overcome unique challenges in biological data and how biological problems can drive advances of computational methodologies.
Date issued
2023-02Department
Massachusetts Institute of Technology. Department of Electrical Engineering and Computer SciencePublisher
Massachusetts Institute of Technology