Harnessing External Data in Public and Private Market Investing
Author(s)
Nahari, Adam D.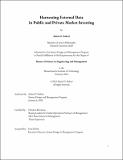
DownloadThesis PDF (2.596Mb)
Advisor
Bertsimas, Dimitris
Terms of use
Metadata
Show full item recordAbstract
Data science is playing an increasingly central role in fundamental investing. This development has been driven by growing awareness of the ways in which external data can be leveraged to create unique insights and support decision-making across the investment lifecycle. Two important trends have undergirded this development. The first has been the rapid growth in the volume and granularity of data available to investors, either publicly or through data vendors. The second has been the growth in technical talent with expertise in such analyses (for example, the relatively recent emergence of roles like data engineers and data scientists), and technologies that make such analyses easier to perform. These trends have enabled investors to examine and evaluate company performance more transparently, and to suggest new insights and levers for improvement.
In this thesis, we propose a generalizable method of evaluating the value of an external data source. We focus specifically on customer review data, and investigate whether customer review data provides a signal of stock price movement that is not accurately or immediately priced by the stock market. We also evaluate whether this signal varies meaningfully across industries, and whether it can be monetized effectively in a trading strategy. We evaluate these questions by examining data from companies’ Google reviews and analyzing the subsequent price action of the respective companies’ shares. Our approach can be easily extended to examine other sources of data and their impact on a number of performance metrics such as earnings per share, free cash flow, revenue, etc.
As mentioned, we seek to determine whether an investor can derive significant value from online Google maps reviews. To test our ideas, we create a backtesting engine that simulates buying or short-selling stocks based on positive or negative changes in review scores, respectively, over varying time horizons, and holding the long or short positions for various lengths of time prior to exiting the position.
Ultimately, we find that a backtesting framework that goes long or short on each stock in a portfolio based on whether the respective company’s rating increased or decreased over a period of time can indeed produce above-market returns at below-market risk.
There is notable variation in returns and standard deviations of returns as the parameters of the score change-period and holding-period change, with the most favorable returns and risk return trade-offs concentrated in strategies that employ longer score change and holding periods. In addition, we find substantial variation in returns across industries, and this also changes considerably from one strategy parameter set to another. Also, the returns from long positions were considerably better than those from short positions, indicating that increases in review scores are much more reliable predictors of increases in stock prices than decreases in review scores are as predictors of declines in stock prices; however, this is a bit misleading since the backtest period was a time of immense growth in equities across the board. We recognize that this analysis leaves ample opportunity to improve upon its strategy and develop further strategies for various other data sources.
Date issued
2023-02Department
System Design and Management Program.Publisher
Massachusetts Institute of Technology