Large-scale programmable silicon photonics for quantum and classical machine learning
Author(s)
Prabhu, Mihika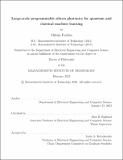
DownloadThesis PDF (45.44Mb)
Advisor
Englund, Dirk R.
Terms of use
Metadata
Show full item recordAbstract
Photonic technologies provide many unique physical advantages including ultra-high bandwidths, energy-efficient operations, and low coupling to environmental noise. Furthermore, recent advances in foundry-based manufacturing platforms have enabled the emerging field of integrated systems photonics. In contrast to their bulk optics counterparts, these systems can co-integrate dense ensembles of active photonic and electronic components on a single wafer with high phase stability and small device footprints. Initial demonstrations of each element in the integrated photonics stack—sources, processors, and detectors—motivate the development of wafer-scale photonic integrated circuit implementations, which are poised to form a key building block for fundamental advancements in computing, communications, and sensing.
The first part of this thesis will discuss the development and early system-level demonstrations of linear programmable nanophotonic processors in the silicon-on-insulator platform for applications in quantum and classical machine learning and information processing. Using our developed processor architecture, we then present a nanophotonic Ising sampler for noise-assisted combinatorial optimization. Subsequently, we present a novel, foundry-compatible platform for integrating telecommunication-wavelength artificial atom quantum emitters directly in silicon photonic circuits. Finally, we report a capacity analysis of a structured interferometric receiver implemented with a silicon photonic processor for detection of optical signals in photon-sparse communication links.
Date issued
2023-02Department
Massachusetts Institute of Technology. Department of Electrical Engineering and Computer SciencePublisher
Massachusetts Institute of Technology