Profile Creation with Topic Modeling and Semantic Analysis from Conversations about COVID-19 among U.S. Older Adults
Author(s)
Le, Joie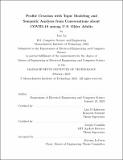
DownloadThesis PDF (2.196Mb)
Advisor
D’Ambrosio, Lisa
Coughlin, Joseph
Terms of use
Metadata
Show full item recordAbstract
Coding of qualitative data in social science research is a process that involves categorizing individual units of data to facilitate analysis. It requires a great deal of manual labor and time to produce codes with high validity and inter-coder reliability.
In an ongoing study, MIT AgeLab researchers analyzed focus group and interview transcripts containing conversations about the impact of the COVID-19 pandemic on Black and white U.S. older adults’ preventive health behavior and healthcare use. To facilitate the qualitative coding process, we propose an approach for automated topic extraction with sentiment analysis using a natural language processing technique known as topic modeling. While automated methods for quantitative data are common, methods for qualitative data, especially focus group text, have not been rigorously explored.
This thesis compares two topic modeling algorithms, LDA and GSDMM, and tests a variety of pseudo-document methods to divide the text transcripts into smaller documents. After the transcripts are split by race, COVID-19 vaccination status, and relationship to a local community, global topics and sentiment-based topics are extracted from the text and labeled by human researchers.
Direct comparisons between profiles within an axis uncover differences warranting further analysis. The results produced from topic modeling can be used to derive an initial codebook pre-coding and push for the investigation of utilizing topic modeling in tandem with human coding during qualitative text analysis.
Date issued
2023-02Department
Massachusetts Institute of Technology. Department of Electrical Engineering and Computer SciencePublisher
Massachusetts Institute of Technology