Combining Masked Autoencoding and Neural Fields for Multi-band Satellite Understanding
Author(s)
Huang, Kuan Wei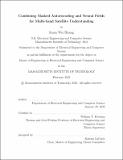
DownloadThesis PDF (2.203Mb)
Advisor
Freeman, William T.
Terms of use
Metadata
Show full item recordAbstract
Multi-spectral satellite remote sensing is a primary way to monitor planet-scale events such as deforestation, land-cover change, fire, and flooding. Unfortunately, incomplete spatial coverage and sparse temporal sampling make it challenging to develop a unified understanding of the environment. We aim to solve these challenges by creating a curated multi-modal satellite remote sensing dataset and presenting a novel architecture that learns a unified representation across large-scale heterogeneous remote sensing data by solving an image completion task. We equip our model with temporal, spectral, and global positioning information in addition to local positional encoding. This allows our algorithm to learn a unified, high-resolution, and time-varying representation across the entire survey area. Unlike the prior work, our architecture does not require data with uniform coverage, temporal resolution, or paired bands, and through prompting, it can act as a method for satellite infilling, temporal prediction, and cross-band translation. We train and evaluate our approach on a multi-modal remote sensing dataset and show that it outperforms baselines across satellite completion and cross-band translation tasks. In addition, we show that the neural feature field learned by our method is more effective than baselines for transfer learning to predict Amazon rainforest deforestation.
Date issued
2023-02Department
Massachusetts Institute of Technology. Department of Electrical Engineering and Computer SciencePublisher
Massachusetts Institute of Technology