Risk-Aware Neural Navigation for Interactive Driving
Author(s)
Jiwani, Suzanna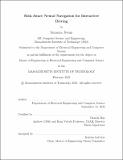
DownloadThesis PDF (2.491Mb)
Advisor
Rus, Daniela
Terms of use
Metadata
Show full item recordAbstract
Safety has been a key goal for autonomous driving since its inception, and we believe recognizing and responding to risk is a key component of safety. In this work, we aim to answer the question, "How can explainable risk representations be used to produce accurate and safe trajectories?". To answer this question, previous work uses risk metrics to formulate an optimization problem. In contrast, our work is based on research showing the usefulness of grids as a representation to generate image-based risk maps through a trained neural network. We propose a novel method of determining risk from a bird’s eye view (BEV) of an autonomous vehicle’s surroundings. Our method consists of (1) a Risk Map Generator, which is trained using a modified loss to encourage recognizing risk associated with nearby agents, (2) value iteration using the risk map to learn a policy, and (3) a Trajectory Sampler, which samples from this policy to generate a trajectory. We uniquely evaluate our planner in an interactive manner, adjusting the surroundings at each time step, and find significant improvements in its overall ability to mimic human driving, with an 86.56% decrease in average displacement error and an 87.72% decrease in the average distance from the goal while maintaining comparable safety statistics when compared with baseline methods. Self ablation also reveals the potential for fine-tuning the behavior of the planner given a designer’s needs.
Date issued
2023-02Department
Massachusetts Institute of Technology. Department of Electrical Engineering and Computer SciencePublisher
Massachusetts Institute of Technology