Essays on Dynamic Supply Chains and Service Delivery Systems
Author(s)
Paine, James Edward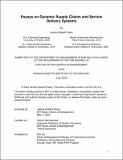
DownloadThesis PDF (8.860Mb)
Advisor
Rahmandad, Hazhir
Terms of use
Metadata
Show full item recordAbstract
The field of Operations Management, and closely related fields of Operations Research and Industrial Engineering, focus intensely on addressing real-world problems associated with the design and management of product and service delivery systems in a human context. System Dynamics is a framework to understand, design for, and manage change emerging from both structural and behavioral features, and is uniquely suited to address policy questions in socio-technical supply chain contexts. Using System Dynamics, Operations Management, and Supply Chain Research methods this work expands on existing toolsets and theory and provides policy insights in dynamic supply chain and service delivery systems.
Chapter 1 presents a methodological contribution to the System Dynamics and Supply Chain Research communities by developing a novel framework for supply chain models by combining three classic methods: co-flow differential equation structures, spot price discovery, and multinomial logistic choice modeling. Chapter 2 applies this framework to build a structural theory explaining the simultaneous surge in food insecurity alongside surges in food surplus and purposeful disposal at the beginning of the COVID-19 pandemic in the United States. Utilizing this structural theory, this chapter further illustrates policies that could help mitigate these stresses. Chapter 3 continues the concepts of managing a behaviorally driven multi-echelon supply subject to shocks. Utilizing a simulated environment, different policy features implied by parallel streams of Operations Management and Supply Chain literature are directly tested. These include policies that range from myopic, limited information decision rules to more modern, but data-intensive machine learning methods.
Date issued
2023-06Department
Sloan School of ManagementPublisher
Massachusetts Institute of Technology