Explicit Regularization for Overparameterized Models
Author(s)
Huang, Tiffany Y.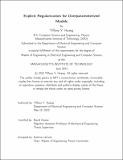
DownloadThesis PDF (706.3Kb)
Advisor
Azizan, Navid
Terms of use
Metadata
Show full item recordAbstract
In many learning problems, it is desirable to incorporate explicit regularization in the objective to avoid overfitting the data. Typically, the regularized objective is solved via weight decay. However, optimizing with weight decay can be challenging because we cannot tell if the solution has reached a global minimum. Further, weight decay can have large run-to-run variations and is sensitive to the choice of regularization hyperparameter. To this end, we propose a new approach to optimize objectives with explicit regularization, called Regularizer Mirror Descent (RMD). In the overparameterized regime, where the number of model parameters exceeds the size of data, RMD provably converges to a point “close” to a minimizer of the regularized objective. Additionally, RMD is computationally efficient and imposes virtually no overhead to standard gradient descent. We observe that RMD is remarkably robust and consistent compared to gradient descent with weight decay despite solving for the same objective. We also illustrate the practical utility of RMD by applying it to learning problems with corrupted labels, where it can match or outperform the state-of-the-art methods without requiring additional hyperparameter tuning or ad-hoc heuristics tailored for this task.
Date issued
2023-06Department
Massachusetts Institute of Technology. Department of Electrical Engineering and Computer SciencePublisher
Massachusetts Institute of Technology