An optimization perspective on log-concave sampling and beyond
Author(s)
Chewi, Sinho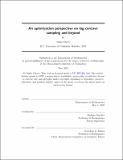
DownloadThesis PDF (6.540Mb)
Advisor
Rigollet, Philippe
Terms of use
Metadata
Show full item recordAbstract
The primary contribution of this thesis is to advance the theory of complexity for sampling from a continuous probability density over R^d. Some highlights include: a new analysis of the proximal sampler, taking inspiration from the proximal point algorithm in optimization; an improved and sharp analysis of the Metropolis-adjusted Langevin algorithm, yielding new state-of-the-art guarantees for high-accuracy log-concave sampling; the first lower bounds for the complexity of log-concave sampling; an analysis of mirror Langevin Monte Carlo for constrained sampling; and the development of a theory of approximate first-order stationarity in non-log-concave sampling.
We further illustrate the main tools in this work—diffusions and Wasserstein gradient flows—through applications to functional inequalities, the entropic barrier, Wasserstein barycenters, variational inference, and diffusion models.
Date issued
2023-06Department
Massachusetts Institute of Technology. Department of MathematicsPublisher
Massachusetts Institute of Technology