Automated Data-driven Algorithm and Mechanism Design in Online Advertising Markets
Author(s)
Liang, Jason Cheuk Nam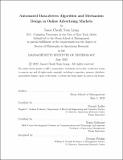
DownloadThesis PDF (4.844Mb)
Advisor
Jaillet, Patrick
Golrezaei, Negin
Terms of use
Metadata
Show full item recordAbstract
Modern automated-bidding (or autobidding) ecosystems have fueled the prevalence of programmatic advertising, which accounts for 90% of total digital ad transaction volume and more than 120$ billion dollar ad spend in 2022. In an autobidding ecosystem, online advertisers convey high-level goals to ad platforms using levers presented by the platforms, and subsequently platforms run automated algorithms to procure ads on advertisers' behalf. While autobidding significantly simplifies and scales up ad procurement processes, it also brings about new challenges: for advertisers, the simplification to ad procurement comes at the cost of information dilution as advertisers no longer have access to granular procurement details; for ad platforms, booming advertiser activities have incentivized growing sophistication in advertising campaign and objectives.
My thesis is dedicated to address two themes from a data-centric perspective: how should advertisers effectively interact with ad platforms in limited information environments? And how should ad platforms design procurement mechanisms to achieve revenue and advertiser welfare goals under complex advertiser objectives and behaviors?
The thesis first addresses the advertisers' problem by exploring how advertisers can utilize levers presented by ad platforms such as budgets or target return-on-investments (ROI) to optimize ad procurement objectives. We analyze the effectiveness of standard platform levers, and then present efficient data-driven algorithms for an advertiser to optimize over lever decisions under limited information, where the procurement algorithm and selling mechanisms in the autobidding ecosystem are treated as a blackbox. Then, the thesis explores an ad platform's problem of designing selling mechanisms against advertisers with complex objectives. In particular, this part of the thesis concerns strategic advertisers who may manipulate selling mechanisms via submitting corrupted information to achieve better long-term rewards, as well as constrained advertisers who are subject to financial restrictions. We first design data-driven pricing algorithms to maximize long-term platform revenue in the presence of different advertiser types. Then, we also investigate how ad platforms can augment standard ad auctions with machine-learned advice to improve worst-case welfare guarantees on the individual advertiser level when advertisers are financially constrained and adopt arbitrary strategies.
Date issued
2023-06Department
Massachusetts Institute of Technology. Operations Research CenterPublisher
Massachusetts Institute of Technology