Global Localization and Guided Relocalization in Unstructured Environments using Semantic Objects
Author(s)
Pedlow, Jacqueline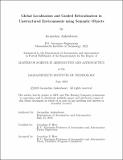
DownloadThesis PDF (15.76Mb)
Advisor
How, Jonathan P.
Terms of use
Metadata
Show full item recordAbstract
This thesis presents a novel framework for global localization and guided relocalization of a vehicle in an unstructured environment. Compared to existing methods, this pipeline does not rely on cues from urban fixtures (e.g., lane markings, buildings), nor does it make assumptions that require the vehicle to be navigating on a road network. Instead, localization is achieved in both urban and non-urban environments by robustly associating and registering the vehicle’s local semantic object map with a compact semantic reference map, potentially built from other viewpoints, time periods, or modalities. Robustness to noise, outliers, and missing objects is achieved through the graph-based data association algorithm. Further, the guided relocalization capability of the pipeline mitigates drift inherent in odometry-based localization after the initial global localization. The pipeline is evaluated on two publicly-available, real-world datasets to demonstrate its effectiveness at global localization in both nonurban and urban environments. The Katwijk Beach Planetary Rover dataset [17] is used to exemplify the pipeline’s ability to perform accurate global localization in unstructured environments at as low as 0.58m accuracy. Demonstrations on the KITTI dataset [15] achieve an average pose error of 3.8m across all 35 localization events on Sequence 00 when localizing in a reference map created from aerial images. Compared to existing works, this pipeline is more generalizable because it can perform global localization in unstructured environments using maps built from different viewpoints and dates.
Date issued
2023-06Department
Massachusetts Institute of Technology. Department of Aeronautics and AstronauticsPublisher
Massachusetts Institute of Technology