A Unified Approach to Controlling Implicit Regularization Using Mirror Descent
Author(s)
Sun, Haoyuan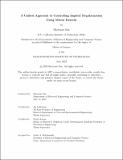
DownloadThesis PDF (1.389Mb)
Advisor
Jadbabaie, Ali
Azizan, Navid
Terms of use
Metadata
Show full item recordAbstract
Inspired by the remarkable performance of deep neural networks, understanding the generalization performance of overparameterized models and the effect of optimization algorithms on it has become an increasingly popular question. In particular, there has been substantial effort to characterize the solutions preferred by the optimization algorithms, such as gradient descent (GD), something referred to as implicit regularization. In particular, it has been argued that GD tends to induce an implicit $\ell_2$-norm regularization in regression and classification problems. Despite significant progress in this space, the implicit bias of various algorithms are either specific to a particular geometry or only exist for a particular class of learning problems, and there is a lack of a general approach for controlling the implicit regularization. To this end, we present a unified approach via mirror descent (MD), which is an important generalization of GD, to control implicit regularization in both regression and classification settings. In particular, we show that MD with a general class of homogeneous potential function converges in direction to a generalized maximum-margin solution for linear classifications problems, thereby answering an open question in the classification setting. Additionally, we show that under suitable conditions, MD can be efficiently implemented with minimal overhead compared to GD and enjoys fast convergence to the maximum-margin solution induced by its implicit bias. Using comprehensive experiments with both linear and deep neural network models, we demonstrate that MD is a versatile method to produce learned models with different regularizers, which in turn lead to different generalization performances.
Date issued
2023-06Department
Massachusetts Institute of Technology. Department of Electrical Engineering and Computer SciencePublisher
Massachusetts Institute of Technology