Atom-by-atom designs of metal oxide catalysts for the oxygen evolution reaction
Author(s)
Lunger, Jaclyn R.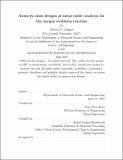
DownloadThesis PDF (40.03Mb)
Advisor
Shao-Horn, Yang
Gomez-Bombarelli, Rafael
Terms of use
Metadata
Show full item recordAbstract
The demand for sustainable energy sources has never been more pressing, and developing innovative, efficient, and eco-friendly energy technologies is essential. The oxygen evolution reaction (OER) holds great promise, as it enables the production of green chemicals, hydrogen gas, and pure metals electrolytically from their oxides, with oxygen as the sole byproduct. However, OER suffers from slow reaction kinetics and a lack of efficient artificial catalysts. In contrast, enzymes such as photosystem II exhibit high efficiency in performing this reaction, a result of millions of years of biological evolution. Thus, the goal of this research is to design optimal metal oxide catalysts for OER that rival the activity of enzymes.
To identify highly efficient catalysts, a systematic approach surpassing current trial-and-error methods is necessary. Complex metal oxides offer a promising class of materials for catalyst design, with billions of potential local atomic structures and active site environments. Therefore, this research combines machine learning, site-level descriptor approaches, and high-throughput virtual screening using density functional theory (DFT) to design optimal metal oxide catalysts for OER. The goal is to identify atom-by-atom design principles that can enhance the efficient production of metals and hydrogen.
In this study, we develop and curate a large open-source dataset of perovskite surfaces with substitutions up to quaternary and facets up to (555) to discover "enzyme-like" active sites. Additionally, we extend our understanding of H-OER to M-OER, a reaction that enables clean metal production analogous to clean hydrogen production, and develop electronic descriptors for screening metals with favorable electrolysis kinetics. Our research offers a comprehensive road-map for the theoretical, atom-by-atom design of catalysts for OER, bridging the gap between current heterogeneous catalysts and enzymes. By utilizing machine learning, DFT, and site-level descriptors, 3 we propose a more targeted and effective approach to catalyst development. Ultimately, this research will contribute to the advancement of sustainable energy technologies and help address the global energy challenge.
Date issued
2023-06Department
Massachusetts Institute of Technology. Department of Materials Science and EngineeringPublisher
Massachusetts Institute of Technology