Improving Segmentation Pipelines for Medical Imaging using Deep Learning
Author(s)
Patel, Jay Biren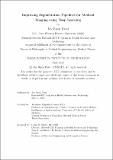
DownloadThesis PDF (20.63Mb)
Advisor
Kalpathy-Cramer, Jayashree
Terms of use
Metadata
Show full item recordAbstract
One of the most important steps in the clinical workflow is the segmentation of medical imaging, which can be used for a variety of clinical decision-making tasks such as disease diagnosis and treatment response evaluation. Manual segmentation of 3D medical imaging (such as computed tomography (CT) or magnetic resonance imaging (MRI)) by a clinical expert can be too time-consuming to be feasible in a routine clinical workflow, and can moreover be susceptible to human errors and inconsistencies. In recent years, deep learning (DL) based methods have exhibited human-level performance for a variety of computer vision tasks, making them an attractive choice for researchers aiming to automate the segmentation of medical imaging. This thesis considers two medical imaging scenarios and examines how fully automatic image segmentation via DL can enhance downstream clinical tasks.
The first scenario evaluates the clinical workflow for diagnosing incidental adrenal masses on CT. Despite standardized reporting systems and strict guidelines for defining an adrenal mass, there exists significant inter-rater variability for this task. To enable objective and reproducible characterization of the adrenal gland, this thesis develops the first DL method for segmentation and classification on CT. Using a large-scale retrospectively acquired dataset, this method is used to identify potential missed detections by radiologists and discuss the clinical implications of this.
The second scenario focuses on the treatment response assessment of metastatic brain tumor patients on MRI. Due to the large number of metastases a patient can have, standard radiographic analyses track only a select few target lesions through the course of therapy in order to assess the efficacy of a treatment. With this paradigm, smaller non-target lesions may be neglected or even missed due to the lack of quantitative emphasis. To that end, a pipeline is developed to automatically segment brain tumor metastases on MRI and output standard response assessment metrics. With the prevalence of longitudinal imaging data available for brain metastases patients, a secondary model is formulated to improve the detection and segmentation of micro-metastases by utilizing known prior time point information.
Date issued
2023-06Department
Harvard-MIT Program in Health Sciences and TechnologyPublisher
Massachusetts Institute of Technology