Transparent Value Alignment: Foundations for Human-Centered Explainable AI in Alignment
Author(s)
Sanneman, Lindsay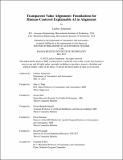
DownloadThesis PDF (2.587Mb)
Advisor
Shah, Julie A.
Terms of use
Metadata
Show full item recordAbstract
Alignment of autonomous agents' values and objectives with those of humans can greatly enhance these agents' ability to act flexibly to safely and reliably meet humans' goals across diverse contexts from space exploration to robotic manufacturing. However, it is often difficult or impossible for humans, both expert and non-expert, to enumerate their objectives comprehensively, accurately, and in forms that are readily usable for agent planning. Value alignment is an open challenge in artificial intelligence that aims to address this problem by enabling agents to infer human goals and values through interaction. Providing humans with direct and explicit feedback about this value learning process through approaches for explainable AI (XAI) can enable humans to more efficiently and effectively teach robots about their goals. In this thesis, we introduce the Transparent Value Alignment (TVA) paradigm which captures this two-way communication and inference process and discuss foundations for the design and evaluation of XAI within this paradigm.
First, we introduce the Situation Awareness Framework for Explainable AI (SAFE-AI), which provides a rigorous approach for comprehensively determining a user's informational needs for their given role and context, identifying which XAI techniques can be applied to meet these needs or gaps in the current state-of-the-art, and evaluating explanation quality. We also review other human factors literature related to cognitive workload and trust in automation and discuss how these constructs additionally inform the design and evaluation of XAI systems.
Next, we propose four metrics for assessing the alignment of reward functions between humans and autonomous agents (i.e. ``reward alignment''). These metrics can be applied to study alignment in scenarios where the human's ground truth reward function is not necessarily directly accessible, as is the case in many real-world settings. We also validate these metrics through a human-subject experiment and a subsequent factor analysis. Findings from this factor analysis indicate the existence of two components comprising the overall reward alignment between humans and agents: feature alignment, which captures how similar a human's reward features and weights are to an agent's, and policy alignment, which captures how similar human and agent policies are for a given reward function.
We also present a series of human-subject experiments which study the efficacy of a broad range of reward explanation techniques across multiple domains. These experiments consider variable reward complexity (defined as the number of features in the reward function), variable task complexity (defined as the number of tasks the human must perform simultaneously when the explanation is provided), and variable team complexity (defined as the number of agents performing the set of required tasks). The results from these experiments together suggest a trade-off between providing users with direct and complete information about the agent's reward function through XAI and increasing their workload. Abstraction-based explanations were a promising approach for balancing these factors, but results also indicated the importance of selecting appropriate abstractions for the particular domain, context, and user. Scenarios with higher team complexities (a larger number of agents) were also subjectively assessed more positively than those with lower team complexities, indicating that in terms of interpretability, simpler decoupled agent plans for larger numbers of agents may be preferable to more complex agent plans for fewer agents.
Finally, we discuss how the TVA problem framing could be applied to real-world domains in the future through a set of case studies. In particular, we highlight findings from a study of key players in the industrial robotics ecosystem in Europe which identified the importance of developing improved robot interfaces and easier-to-program systems for robotics in manufacturing. We also discuss the applicability of TVA to space mission planning, which is informed by observations of the tactical planning process for the Mars Curiosity rover at the NASA Jet Propulsion Laboratory (JPL). Lastly, we discuss how TVA could be applied to human-autonomy teaming scenarios such as search-and-rescue mission planning.
Date issued
2023-06Department
Massachusetts Institute of Technology. Department of Aeronautics and AstronauticsPublisher
Massachusetts Institute of Technology