Unsupervised Representation Learning from Intravascular Ultrasound Videos
Author(s)
Jain, Lay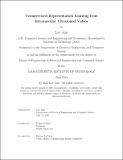
DownloadThesis PDF (6.453Mb)
Advisor
Golland, Polina
Terms of use
Metadata
Show full item recordAbstract
Vascular diseases such as atherosclerosis are a leading cause of mortality and morbidity worldwide. Intravascular Ultrasound (IVUS) is an imaging technology that has the distinctive ability to offer real-time endovascular information of the coronary vasculature. However, its low signal-to-noise ratio, low data availability, and numerous artifacts make it challenging to use both for humans and automated methods. This work explores the use of representation learning and de-noising techniques to address these challenges and aid in the diagnosis of vascular diseases. We test our methods on the task of stent malapposition detection, where naive approaches fail discouragingly. We improve the naive baseline accuracy by 16%. In addition, we develop a deep learning approach for real-time stabilization of the IVUS videos, which performs registration 20-fold faster than the classical ANTs approach. Our results demonstrate the importance of incorporating domain knowledge in performance improvement while still indicating the limitations of current systems for achieving clinically ready performance.
Date issued
2023-06Department
Massachusetts Institute of Technology. Department of Electrical Engineering and Computer SciencePublisher
Massachusetts Institute of Technology