Predicting and Preventing Unsafe Events at an Enterprise
Author(s)
Ukaire, Onyinyechi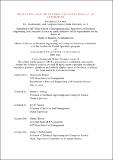
DownloadThesis PDF (4.649Mb)
Advisor
Boning, Duane S.
Welsch, Roy E.
Terms of use
Metadata
Show full item recordAbstract
Amgen Incorporated, like many organizations with manufacturing operations, has undesirable incidents. Corporate workers trip and fall. Contractors get cuts that require first aid. Shop floor operators can be injured by automated equipment, and so on. Seeking to become a leader in environmental health and safety, more so than avoiding painful costs related to work-related injuries, Amgen wants to curb and ultimately reduce its unwanted events to zero. To do so, a dominant approach is to build a model that predicts undesirable incidents. If predicted, then it can be prevented. Previous studies predicting safety relied on incidence rate per worker, medical costs, or days away from work — variables biased to staff reporting practices. The availability of standardized, routinely, and automatically collected data on work equipment, work orders, human resources allow for the hypothesis that operating parameters are indicative of unwanted events. We find that machine and work order variables are poor indicators of the frequency of unsafe incidents. A reason for this unexpected finding is that the company has reduced machine generated errors to the extent that they are no longer the primary drivers of unsafe events in the system. In checking for alternative drivers of systemic safety, we find human factors promising. Even when a predictive model is in effect, we demonstrate the need to develop organizational capabilities to ensure that safety systems continuously improve. We argue for a new approach that puts standardization of incident documenting, data engineering, and performance metrics reporting at the core. Given ample opportunities for evaluating safety systems, we are confident that those variabilities that result in accidents can be subdued for Amgen and many industrial companies.
Date issued
2023-06Department
Massachusetts Institute of Technology. Department of Electrical Engineering and Computer Science; Sloan School of ManagementPublisher
Massachusetts Institute of Technology