Neural Networks on Eigenvector Data
Author(s)
Lim, Derek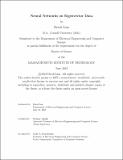
DownloadThesis PDF (8.677Mb)
Advisor
Jegelka, Stefanie
Terms of use
Metadata
Show full item recordAbstract
The need to process eigenvectors derived from data arises across numerous domains in computing and the sciences. However, eigenvectors differ from other types of data, as they have particular symmetries; for any eigenvector of a matrix, the negation of that vector is also an eigenvector of the same eigenvalue, so there are sign symmetries. There are also more general continuous basis symmetries in higher dimensional eigenspaces. In this thesis, we present the first neural networks that process eigenvector input while respecting these symmetries. We build neural networks that are invariant to sign and basis symmetries as well as neural networks that are equivariant to sign symmetries. Under certain conditions, these networks are provably universal — they can approximate any continuous functions with the desired invariances. When used with Laplacian eigenvectors, our invariant neural networks are provably powerful for graph representation learning, as they can approximate several classes of important functions on graphs. Our networks empirically improve machine learning models with eigenvectors, in tasks including molecular graph regression, learning expressive graph representations, and learning neural fields on triangle meshes.
Date issued
2023-06Department
Massachusetts Institute of Technology. Department of Electrical Engineering and Computer SciencePublisher
Massachusetts Institute of Technology