Multimodality: Models, Algorithms, and Applications
Author(s)
Boussioux, Léonard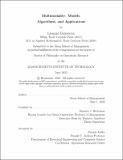
DownloadThesis PDF (78.59Mb)
Advisor
Bertsimas, Dimitris J.
Terms of use
Metadata
Show full item recordAbstract
As global sustainability challenges intensify, the demand for innovative, interdisciplinary solutions that leverage diverse data sources and analytical methods is surging. We investigate combining operations research and artificial intelligence to address urgent sustainability and healthcare concerns by developing adaptable, universally applicable frameworks.
This thesis delves into multimodality by using simultaneously distinct data types, such as tables, images, time series, and free text. We formulate versatile methodologies that can be applied to various tasks, from tropical cyclone forecasting and biodiversity tracking to healthcare operations, with minimal adaptation. We mimic human abilities to comprehend and connect different data types by incorporating artificial intelligence and optimization in data-driven strategies. Our contributions include the development of generalizable data pre-processing, feature extraction, and data fusion pipelines that facilitate large-scale multimodal data processing in complex real-world scenarios. Notably, our tropical cyclone forecasting models demonstrate performance comparable to the US National Hurricane Center's top models for 24-hour intensity and track forecasts.
Moreover, we build integrated predictive-to-prescriptive data-driven frameworks connecting operations research and artificial intelligence. In support of multimodality, we introduce innovative tools that ensure model reliability and performance in critical situations. We explore adaptive robust ensemble modeling to augment planning and decision-making under uncertainty. Our predictive and prescriptive models have been effectively implemented in factories, museums, and hospitals to tackle sustainability and public health issues, including air pollution management, ecosystem preservation, and rare tumor segmentation. Our pollution management models considerably reduced harmful emissions at the OCP Safi Site, Morocco's largest chemical industrial plant, while cutting unnecessary costs. In addition, our tumor segmentation models match medical doctors' expertise while offering substantial time savings.
Date issued
2023-06Department
Massachusetts Institute of Technology. Operations Research CenterPublisher
Massachusetts Institute of Technology