AI-Based Speech Assessment of Cognitive Impairment Disorders
Author(s)
Haulcy, R'mani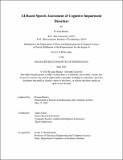
DownloadThesis PDF (6.312Mb)
Advisor
Glass, James
Terms of use
Metadata
Show full item recordAbstract
Previous research has shown that speech can be used to detect cognitive impairment in patients with dementia and other neurodegenerative diseases. These diseases produce cognitive deficits that lead to changes in the acoustic and linguistic content of the speech produced by the patients.
In this thesis, we analyze the speech of subjects with Alzheimer’s Disease (AD), Frontotemporal Dementia (FTD), and Primary Progressive Aphasia (PPA). We show that AD subjects can be distinguished from healthy controls with 85.4% accuracy and that the Mini- Mental State Examination scores of the subjects can be predicted with a root mean squared error of 4.56, using sentence embeddings. We present the Crowdsourced Language Assessment Corpus (CLAC), a corpus that we created to provide the community with a collection of audio samples from various speakers that can be used to learn a general representation for speech from healthy subjects, as well as complement other health-related speech datasets.
We present a novel, language-agnostic approach for measuring the quality of repetition in a recording, a method that was inspired by the need to automatically quantify the impaired repetition abilities that characterize the speech of people with the logopenic variant of PPA (lvPPA). A subset of the CLAC corpus was used as healthy controls and we demonstrated the feasibility of our approach by using it to distinguish between healthy and lvPPA speakers with impaired repetition with 85.7% accuracy. Lastly, we compare standard linguistic features to more advanced sentence embeddings by using a variety of feature extraction methods to extract features from picture description and monologue data for four different FTD/PPA variants. We show that all variants can be distinguished from healthy controls with >= 90% accuracy using transformer-based sentence embeddings.
We hope that the work presented in this thesis will contribute to the goal of using artificial intelligence to improve human health, clinical trial design, and drug development.
Date issued
2023-06Department
Massachusetts Institute of Technology. Department of Electrical Engineering and Computer SciencePublisher
Massachusetts Institute of Technology