Comparing Phylogenetic and Deep Learning Methods to Predict Seed Dispersal Mode
Author(s)
Xu, Haodi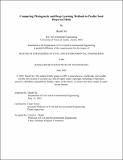
DownloadThesis PDF (7.212Mb)
Advisor
Terrer, César
Terms of use
Metadata
Show full item recordAbstract
Increasing tree cover is a promising natural climate solution to reduce carbon under the pressing global warming. Seed dispersal is a key process in natural forest regrowth, where seeds are moved away from parent plants to establish new growth. Dispersal modes include biotic and abiotic methods, and vary depending on traits such as seed shape, size, and color. However, globally, data on seed dispersal modes of plant species is limited, hindering our understanding of the importance of wild animals in increasing tree cover and their role in carbon sequestration. The research goal of this study is to find a method to predict unknown seed dispersal modes with high accuracy by comparing a novel deep learning method with a typical phylogenetic imputation method. Here we show that the phylogenetic imputation method performed better than deep learning methods in predicting biotic seed dispersal mode. However, we also found that the deep learning methods demonstrate great potential in learning from community science photographs, despite their underperformance in this study. Furthermore, the study shows that incorporating a feature-extraction model could improve predictions of a single CNN model, highlighting the potential for future studies to include more models for better predictions of seed dispersal modes. We anticipate that the problems and potential improvements identified in this study relating to the deep learning method will serve as a starting point for further model development to predict the seed dispersal mode of unknown species with greater accuracy. This could involve applying multiple models, incorporating phylogenetic information with deep learning models, and including additional features. Accurately understanding how different plant species are dispersed can help scientists better predict future forest dynamics and carbon storage capacity, which is critical for studying future climate change and developing effective climate change mitigation strategies.
Date issued
2023-06Department
Massachusetts Institute of Technology. Department of Civil and Environmental EngineeringPublisher
Massachusetts Institute of Technology