Capturing Tacit Knowledge of Experts through the Study of Visual Attention: Applications for Human Expertise and AI
Author(s)
Armengol Urpí, Àlex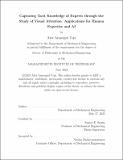
DownloadThesis PDF (25.31Mb)
Advisor
Sarma, Sanjay E.
Terms of use
Metadata
Show full item recordAbstract
Tacit or implicit knowledge is know-how that humans cannot convey explicitly; it is difficult to verbalize, and hence, it is challenging to transfer to others in words. Tacit knowledge is usually gained from experience and internalized unconsciously through implicit learning. Since tacit knowledge is not consciously accessible, it is commonly seen as a “mysterious" part of expertise that can only be transferred from one person to another through close interaction, coaching, mentoring, and observation of expert behavior. Examples of daily activities based on tacit knowledge include riding a bike, recognizing a face, writing a persuasive thesis or speaking a native language. This thesis explores new methods for tacit knowledge extraction using visual attention-based human-computer interfaces.
Earlier studies suggest that eye gaze is particularly suited for studying the unconscious component of expertise. For this reason, this research focuses on developing new interfaces that track the visual attention of experts while performing tasks in which they excel. This thesis is divided into two main sections. In the first part, we develop novel human-computer interfaces suited to track visual attention and that enhance existing interfaces based on gaze tracking alone. We do this by exploiting brain activity in addition to eye gaze. First, we leverage neural mechanisms of visual attention to improve the accuracy of a commercial eye tracker through the analysis of electroencephalography (EEG) waves. Our hybrid system combines EEG and eye-tracking modalities to overcome the accuracy limitations of the gaze tracker alone. We integrate EEG and gaze data to efficiently exploit their complementary strengths by driving a Bayesian probabilistic decoder that estimates the region in the visual field gazed at by the user. This demonstrates that the intrinsic accuracy limitations of camera-based eye trackers can be corrected with the integration of EEG data. Then, we show why visual attention and gaze can be decoupled by developing an interface that tracks peripheral attention using EEG waves. Our novel approach can detect peripheral (or covert) spatial attention by using single-frequency phase-coded stimuli that elicit the corresponding Steady-State Visually Evoked Potentials (SSVEPs). This opens opportunities for attention-tracking applications with largely increased number of targets in the visual field.
In the second part of this thesis, we exploit our previously developed interfaces to track the visual attention of experts performing image classification tasks. First, we create images with a hidden asymmetry that is not consciously (or explicitly) recognized by the experts. However, their visual attention patterns reveal that the asymmetry is unconsciously internalized because the attention metrics are skewed towards the image regions most relevant for categorization. This demonstrates that we can capture insights about experts' tacit knowledge by tracking their visual attention. We then show that the expertise of subjects who receive feedback extracted from their own attention patterns is significantly enhanced compared to subjects who did not. We refer to this as cognitive reinforcement. This research opens the door to new ways in which human expertise can be enhanced, exploited, and transferred. Finally, we utilize human attention maps captured during image exploration and labeling to feed a CNN-based image classification model. We demonstrate that when few images are available for training, the model fed with human attention maps, in addition to images and labels, significantly outperforms the baseline model. These results illustrate that experts' tacit knowledge can be exploited to enhance the performance of human experts as well as AI systems.
Date issued
2023-06Department
Massachusetts Institute of Technology. Department of Mechanical EngineeringPublisher
Massachusetts Institute of Technology