Understanding Soil Carbon Signatures from Hyperspectral Reflectance Data using Spectral Unmixing
Author(s)
Zeng, Xinyi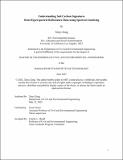
DownloadThesis PDF (11.34Mb)
Advisor
Terrer, César
Terms of use
Metadata
Show full item recordAbstract
Soil carbon stocks have been depleted mainly due to human activities, and there is a potential for soil carbon sequestration through regenerative agricultural practices, forest restoration, and similar interventions. The traditional laboratory treatments of soil samples provide ground-truth soil carbon content, but they are usually costly, time-consuming, and provide only one-time measurements with limited spatial coverage and resolution. The accelerating development of soil spectroscopy offers an opportunity for cheaper, more immediate, and continuous measurements of soil carbon content. Moreover, the recent advancement of hyperspectral imagers has significantly increased spectral resolution, allowing more granular information to be captured. These devices offer a potentially more accurate methodology to quantify and monitor soil properties globally. Nevertheless, there is no consensus on the optimal practices for soil carbon content estimation using hyperspectral reflectance data. Therefore, this thesis tests whether it is feasible to leverage spectral linear mixing models to decompose soil hyperspectral reflectance data into interpretable soil component spectral signatures and abundances. The results demonstrate that the proposed spectral linear mixing model can predict soil organic carbon (SOC) spectral signature and mass abundance with a nearly 0 average bias. However, biases can still be significant for certain spectra. To reduce these biases, it is essential to characterize the problem more effectively. Dedicated soil spectral data collection efforts designed explicitly for unmixing applications could enhance the quality of the results and contribute to a more comprehensive understanding of SOC spectrum and abundance. These findings motivate further development and refinement of spectral mixing models, as well as research into the application of hyperspectral reflectance data to soil property analysis.
Date issued
2023-06Department
Massachusetts Institute of Technology. Department of Civil and Environmental EngineeringPublisher
Massachusetts Institute of Technology