Coarse-grained models for prediction, uncertainty quantification, and extreme event statistics of turbulent flows in engineering and geophysical settings using physics-consistent data-driven closures
Author(s)
Charalampopoulos, Alexis-Tzianni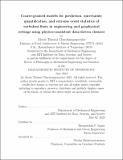
DownloadThesis PDF (27.67Mb)
Advisor
Sapsis, Themistoklis P.
Terms of use
Metadata
Show full item recordAbstract
Modeling and analysis of turbulent fluid flows remains one of the challenging areas of fluid mechanics where integration of the full equations is associated with extreme computational cost, while their simplification inevitably introduces important model errors. In this work we are aiming to answer three questions: How can we use the governing equations and available datasets to formulate physics-constrained data- driven closures that will provide accurate coarse-grained evolution equations? Can we formulate the corresponding physics-constrained closures for the quantification of uncertainty? Can high-order statistics and statistics of extreme events be computed from data-augmented coarse-scale or reduced-order models? These questions are motivated by real-world problems, such as multiphase fluid flows as well as climate modeling.
To address these questions we adopt a statistical formulation of the governing equations and employ machine-learning ideas to formulate, physics informed, non- local in space and time closure schemes for turbulent, possibly multi-phase, fluid flows found in engineering and geophysical settings. The generic form of the systems we study includes a linear operator, external forcing and a bi-linear, energy-preserving operator. We use the predictions of neural networks to integrate over a coarse grid the evolution of turbulent anisotropic fluid flows on which bubbles that act as passive inertial tracers are being transported.
To answer the first question we develop closures for the mean flow field that we complement with physical constraints, which follow from the energy-preserving character of the bi-linear operator. Next, we proceed with the second question by formulating the second-order moment equations for which we also derive data-driven closures that we complement with appropriate physical constraints. We utilize recurrent and convolutional layers to capture both temporal and spatially non-local effects. The addition of the physical constraints not only improves the performance of the resulted closures but also stabilizes the coarse-grained equations in cases that are otherwise unstable. The approach is tested both for closing the mean equation and the covariance evolution equation in a second-order statistical framework. The closure schemes for turbulent fluid flows are complemented with a method that aims to predict high-order moments of bubble cluster deformation from second-order statistics. This is achieved with the introduction of a hybrid quadrature method of moments appropriate for finite-dimensional dynamical systems. We demonstrate the resulted closures and assess the generalizability properties in different Reynolds and flow configurations.
To answer the third question we machine learn non-intrusive correction operators that use as input imperfect, i.e. coarse-scale, climate model outputs that typically have discrepancies due to low resolution. An important challenge in this case is the chaotic character of the underlying dynamics which makes the machine learning of the correction operator an intractable task. To overcome this obstacle we design a new approach based on nudging, a popular method for data-assimilation in geophysical modeling, to create consistent training datasets between the input and the output of the correction operator. We illustrate that the resulted non-intrusive correction operator is able to improve inaccuracies of the coarse-scale model attractor making it consistent with the target attractor. This allow us to obtain extreme event statistics of climate extreme events such as hurricanes and atmospheric rivers, using as input only inexpensive coarse-scale simulations. To this end, the introduced approach paves the way for the parsimonious study of climate-change scenarios and the effectiveness of possible measures or policies.
Date issued
2023-06Department
Massachusetts Institute of Technology. Department of Mechanical Engineering; Massachusetts Institute of Technology. Institute for Data, Systems, and SocietyPublisher
Massachusetts Institute of Technology