Lifelong Personalization for Social Robot Learning Companions: Interactive Student Modeling Across Tasks and Over Time
Author(s)
Spaulding, Samuel Lee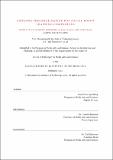
DownloadThesis PDF (16.54Mb)
Advisor
Breazeal, Cynthia
Terms of use
Metadata
Show full item recordAbstract
Early language and literacy skills are important foundations for learning and form the basis of later academic success. Motivated by a growing scientific consensus that language learning requires engaging students cognitively, affectively, and socially, this thesis advances work to develop “social robot learning companions" that engage with and adapt to students across different language/literacy tasks to provide long- term, scalable, and personalized learning assistance. Personalized student modeling helps promote learning and engagement, but sophisticated modeling relies heavily on student interaction data. In order to elicit useful amounts of personalized student data, researchers have increasingly employed “long-term" interaction designs, which occur over distinct sessions at different times.
This thesis broadens the scope of single-task “long-term personalization" to “multi-task personalization" across different tasks. Both “long-term" and “multi-task" personalized interaction designs are mirrored by an associated shift in algorithm and model design: continual learning, which accounts for the temporal sequence in which data is received, and transfer learning, which accounts for the task in which data originates, using data from a ‘source’ task to learn a model in a different ‘target’ task. The combination of these paradigms, which I call “lifelong personalization" could lead to flexible personalized models that can better adapt to individuals over time and across tasks.
This thesis is a presentation and evaluation of continual and transfer learning methods, focusing on their impact on accuracy and data efficiency of personalized student models, and on student learning and engagement. To facilitate this research, I have developed a unified robotic game system for studying lifelong personalization over two different educational games, each emphasizing certain language and literacy skills. The robot’s behavior in each game is backed by a flexible Gaussian Process-based approach for rapidly learning student models from interactive play in each game, and a method for transferring each game’s learned student model to the other via a novel instance-weighting protocol based on task similarity. By evaluating new methods for flexible, adaptive student personalization within a suite of custom-designed games for promoting students’ language/literacy skills, this thesis contributes both algorithmic and human-centered insights for the future of educational human-robot interactions.
Date issued
2022-09Department
Program in Media Arts and Sciences (Massachusetts Institute of Technology)Publisher
Massachusetts Institute of Technology