Determinants and Interventions for Physical Activity Adherence During COVID-19: A Global Study Using Machine Learning Approach
Author(s)
Chai, Yuchen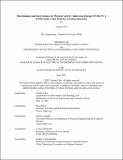
DownloadThesis PDF (3.056Mb)
Advisor
Zheng, Siqi
Jegelka, Stefanie
Terms of use
Metadata
Show full item recordAbstract
Physical activity (PA) is crucial for maintaining both physical and mental health in urban and regional settings. However, public health hazards, such as pandemics, extreme temperatures, and air pollution, pose challenges for PA adherence due to voluntary or mandatory self-protection measures and the closure of exercise facilities in cities. Existing research on urban health resilience during crises primarily depends on small-scale exercise surveys and fails to consider the multifaceted determinants of exercise, including personal habits, social networks, and local policy or built environments. In this project, I use COVID-19 as a case study to systematically investigate the drivers of unequal PA adherence and identify opportunities for timely personalized interventions. First, I collect the universe of exercise records for 30 million individuals across more than 200 countries from Strava. Then, I develop advanced neural network methods to automate the identification of PA adherence prior and during the pandemic based on personal exercise habits and social network interactions, achieving accuracy rates of 89.9% and 82.1% respectively. Lastly, I integrate an explainable neural network approach with econometric analysis to reveal the impact of city-level policies, socio-demographics, and built environment factors on PA inequality. My findings suggest that regions worldwide experience significant PA shocks at the onset of the pandemic, particular during lockdown periods, yet followed by a positive rebound in the long term. Males and urbanites in less developed regions tend to experience more negative PA shocks during the COVID-19, likely moderated by exercise preferences and the availability of outdoor sports amenities. Social connectivity also plays a vital role in promoting PA adherence during crises. This study advances the field by combining large-scale digital data with machine-learning to provide in-time prediction of PA adherence and map its complex determinants. My thesis thus provides direct evidence-based support for multi-layered PA interventions from personal nudges, social networks, and city planning perspectives during public health crises.
Date issued
2023-06Department
Massachusetts Institute of Technology. Department of Electrical Engineering and Computer Science; Massachusetts Institute of Technology. Department of Urban Studies and PlanningPublisher
Massachusetts Institute of Technology