Application of deep learning to land cover classification: practical issues and strategies
Author(s)
Fang, Ruoming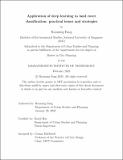
DownloadThesis PDF (17.17Mb)
Advisor
Hsu, David
Terms of use
Metadata
Show full item recordAbstract
Land Use and Land Cover (LULC) change is a process of essential importance to urban studies and planning. Large-scale databases provide comprehensive records, but in many circumstances, they need to be supplemented or substituted by alternative data sources. The advent of deep learning provides an efficient, low-cost data generation method in which a trained deep neural network (DNN) segments satellite images to classify land cover; in recent years, multiple models have been proposed and tested on satellite imagery. This study takes a practically oriented approach, in which we train a classic convolutional neural network (CNN) model on a novel labeled image dataset, then use the model to segment Sentinel-2 satellite images and classify the land cover of Massachusetts in 2019. While the model performs very well in classifying land covers at a broad level, the discrepancies between model predictions and reference data increase in distinguishing more nuanced land features due to many localized factors. In addition, model training and classification are highly sensitive to several issues specific to remote sensing data, such as defects in images and distribution shifts. We devise multiple empirical strategies to address these issues, including a progressive technique to select high-quality data samples from the imperfect dataset and the selection of normalization parameters to reduce the impact of covariate shifts. We contend that good models alone are insufficient to drive successful LULC mapping on remote sensing imagery; sound data engineering also plays a crucial role. Lastly, we explore potential improvements in the field that can benefit future applications.
Date issued
2023-02Department
Massachusetts Institute of Technology. Department of Urban Studies and PlanningPublisher
Massachusetts Institute of Technology