The Trainability and Expressivity of Quantum Machine Learning Models
Author(s)
Anschuetz, Eric R.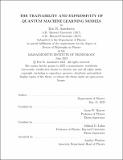
DownloadThesis PDF (4.211Mb)
Advisor
Harrow, Aram W.
Lukin, Mikhail D.
Terms of use
Metadata
Show full item recordAbstract
Research over the last few decades has provided more and more evidence that precise control of many-body quantum systems yields a method of computation more powerful than what is achievable using conventional models of computation. This culminated in recent years with experimental demonstrations on quantum devices of computational tasks on the verge of classical intractability.
These current generation quantum devices are, however, too noisy and small to perform any means of error correction. These limitations motivate the study of hybrid quantum-classical algorithms as potential practical use-cases of these devices in the near-term. This thesis is concerned with studying potential use-cases of these hybrid algorithms, determining limitations of algorithms constructed via this framework, and giving provable guarantees on the performance of such algorithms. Specifically:
1. We consider quantum-classical hybrid algorithms which are framed as optimization problems with quantum-evaluated loss functions. We show that when the operations implemented on the quantum device are drawn from a certain problem-independent distribution, the loss landscapes (in expectation) exhibit a phase transition in trainability. We argue that the trainable phase is typically unachievable, and thus that such algorithms are not practical to implement.
2. We sharpen these arguments and show similar behavior for local, shallow, variational quantum algorithms. We also study the impact of noise on such algorithms when they are in the trainable phase, and show that such noise is capable of making otherwise trainable algorithms untrainable in a statistical query setting.
3. We give efficient classical algorithms to simulate certain variational quantum algorithms that circumvented the assumptions of our previous results and were known to be trainable, demonstrating that care must be taken to strike a balance between quantum implementability and classical intractability.
4. We prove unconditionally that certain quantum neural networks are more expressive than a wide class of classical neural networks and demonstrate that quantum contextuality is the resource for this separation. We also give arguments (along with numerical evidence) that such models are efficiently trainable, thus showing that there exists a regime where hybrid quantum-classical algorithms outperform their purely classical counterparts.
Date issued
2023-06Department
Massachusetts Institute of Technology. Department of PhysicsPublisher
Massachusetts Institute of Technology