Information-theoretic Algorithms for Model-free Reinforcement Learning
Author(s)
Wu, Farrell Eldrian S.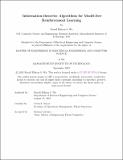
DownloadThesis PDF (423.4Kb)
Advisor
Farias, Vivek F.
Terms of use
Metadata
Show full item recordAbstract
In this work, we propose a model-free reinforcement learning algorithm for infinte-horizon, average-reward decision processes where the transition function has a finite yet unknown dependence on history, and where the induced Markov Decision Process is assumed to be weakly communicating. This algorithm combines the Lempel-Ziv (LZ) parsing tree structure for states introduced in [4] together with the optimistic Q-learning approach in [9]. We mathematically analyze the algorithm towards showing sublinear regret, providing major steps towards the proof of such. In doing so, we reduce the proof to showing sub-linearity of a key quantity related to the sum of an uncertainty metric at each step. Simulations of the algorithm will be done in a later work.
Date issued
2023-09Department
Massachusetts Institute of Technology. Department of Electrical Engineering and Computer SciencePublisher
Massachusetts Institute of Technology