Upper and Lower Bounds for Sampling
Author(s)
Lu, Chen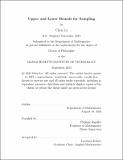
DownloadThesis PDF (5.070Mb)
Advisor
Rigollet, Philippe
Terms of use
Metadata
Show full item recordAbstract
This thesis studies the problem of drawing samples from a probability distribution. Despite the prevalence of sampling problems in applications, the quantitative behavior of sampling algorithms remains poorly understood. This thesis contributes to the theoretical understanding of sampling by giving upper bounds and more importantly lower bounds for various sampling algorithms and problem classes. On the upper bound side, we propose new sampling algorithms, motivated by the perspective of sampling as optimization [JKO98], and give convergence guarantees for them. We also obtain state-of-the-art convergence results for the popular Metopolis-Adjusted Langevin Algorithm. On the lower bound side, we establish the query complexity for strongly log-concave sampling in all constant dimensions. Our lower bounds rely on simple geometric constructions, which can hopefully be of aid to similar results in high dimensions.
Date issued
2023-09Department
Massachusetts Institute of Technology. Department of MathematicsPublisher
Massachusetts Institute of Technology