Stochastic Sea Ice Modeling with the Dynamically Orthogonal Equations
Author(s)
Suresh Babu, Anantha Narayanan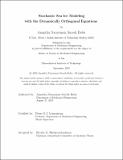
DownloadThesis PDF (14.24Mb)
Advisor
Lermusiaux, Pierre F.J.
Terms of use
Metadata
Show full item recordAbstract
Accurate numerical models are essential to predict the complex evolution of rapidly changing sea ice conditions and study impacts on climate and navigation. However, sea ice models contain uncertainties associated with initial conditions and forcing (wind, ocean), as well as with parameter values, functional forms of the constitutive relations, and state variables themselves, all of which limit predictive capabilities. Due to the multiple types and scales of sea ice and the complex nonlinear mechanics and high dimensionality of differential equations, efficient ocean and sea ice probabilistic modeling, Bayesian inversion, and machine learning are challenging. In this work, we implement a deterministic 2D viscoplastic sea ice solver and derive and implement new sea ice probabilistic models based on the dynamically orthogonal (DO) equations.
We focus on the stochastic two-dimensional sea ice momentum equations with nonlinear viscoplastic constitutive law. We first implement and verify a deterministic 2D viscoplastic sea ice solver. Next, we derive the new stochastic Sea Ice Dynamically Orthogonal equations and develop numerical schemes for their solution. These equations and schemes preserve nonlinearities in the underlying spatiotemporal dynamics and evolve the non-Gaussianity of the statistics. We evaluate and illustrate the new stochastic sea ice modeling and schemes using idealized stochastic test cases. We employ two stochastic test cases with different types of sea ice: ice sheets and frozen ice cover with uncertain initial velocities. We showcase the ability to evolve non-Gaussian statistics and capture complex nonlinear dynamics efficiently. We study the convergence to the physical discretization, and stochastic convergence to the stochastic subspace size and coefficient samples. Finally, we assess and show significant computational and memory efficiency compared to the direct Monte Carlo method.
Date issued
2023-09Department
Massachusetts Institute of Technology. Department of Mechanical EngineeringPublisher
Massachusetts Institute of Technology