Conformal Methods for Efficient and Reliable Deep Learning
Author(s)
Fisch, Adam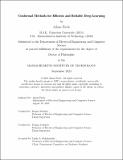
DownloadThesis PDF (10.01Mb)
Advisor
Barzilay, Regina
Jaakkola, Tommi
Terms of use
Metadata
Show full item recordAbstract
Deep learning has seen exciting progress over the last decade. As large foundation models continue to evolve and be deployed into real-life applications, an important question to ask is how we can make these expensive, inscrutable models more efficient and reliable. In this thesis, we present a number of fundamental techniques for building and deploying effective deep learning systems that are broadly based on conformal prediction, a model-agnostic and distribution-free uncertainty estimation framework. We develop both theory and practice for leveraging uncertainty estimation to build adaptive models that are cheaper to run, have desirable performance guarantees, and are general enough to work well in many real-world scenarios. Empirically, we primarily focus on natural language processing (NLP) applications, together with substantial extensions to tasks in computer vision, drug discovery, and medicine.
Date issued
2023-09Department
Massachusetts Institute of Technology. Department of Electrical Engineering and Computer SciencePublisher
Massachusetts Institute of Technology